Software platforms dedicated to literature search and review
The common theme for popular commercial AI applications that explicitly pitch themselves towards ‘research’ is that they integrate LLM technology with search technology to accelerate and, to a limited extent, enhance literature searching and (limited) reviewing. This reflects the misleading lay perception of research which is often thought of as primarily just ‘reading’. There are some literature mapping tools (like Research Rabbit (free), SciSpace (free + paid), Semantic Scholar (free) and Connected Papers (free + paid)) that don’t use LLMs (but use limited semantic NLP) to enhance searches but are nonetheless very useful for building up literature collections. In fact they are arguably better for systematic reviews where time saving is less important than breadth of coverage.
The most popular tools that use advanced generative AI to support literature review are Undermind, Elicit and Scite AI. As with any software application, while they fundamentally perform similar functions they each have distinct value propositions and interfaces that will be more useful for some researchers and contexts.
As of 2025, the leading AI tool for literature discovery is Undermind. Its primary distinguishing feature is a high quality searching and ranking methodology (read their white paper comparing to Google Scholar) . It also keeps the AI summary content minimal, which is useful to incentivise actually opening the sources directly, as opposed to other tools which provide more (but inevitably unreliable) AI summaries that can look reassuring and lead to users basing decisions on the AI content rather than the paper itself. Undermind pitches itself as a 'by researchers, for researchers' platform and the less flashy interface dominated by dense, relevant links to the sources suggests this is sincere. Example of its search results:
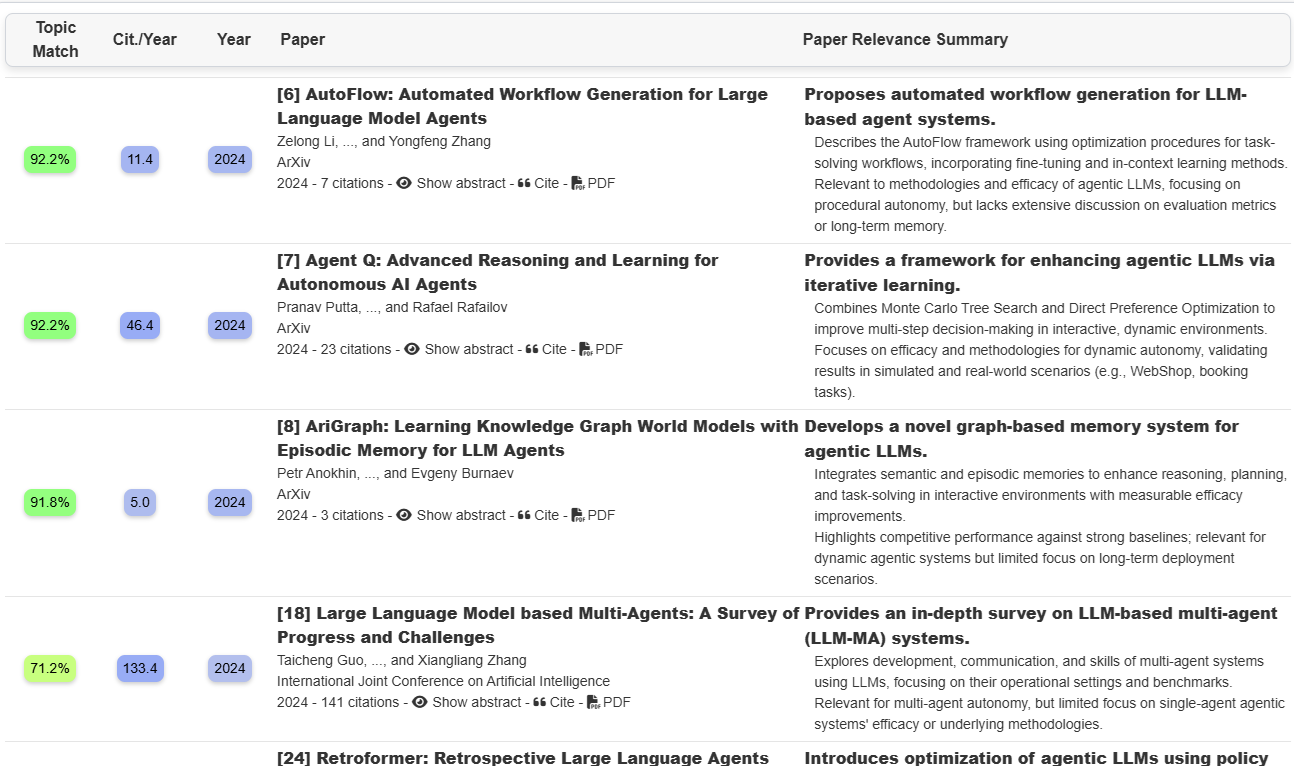
Example of output from the general chat following results retrieval:
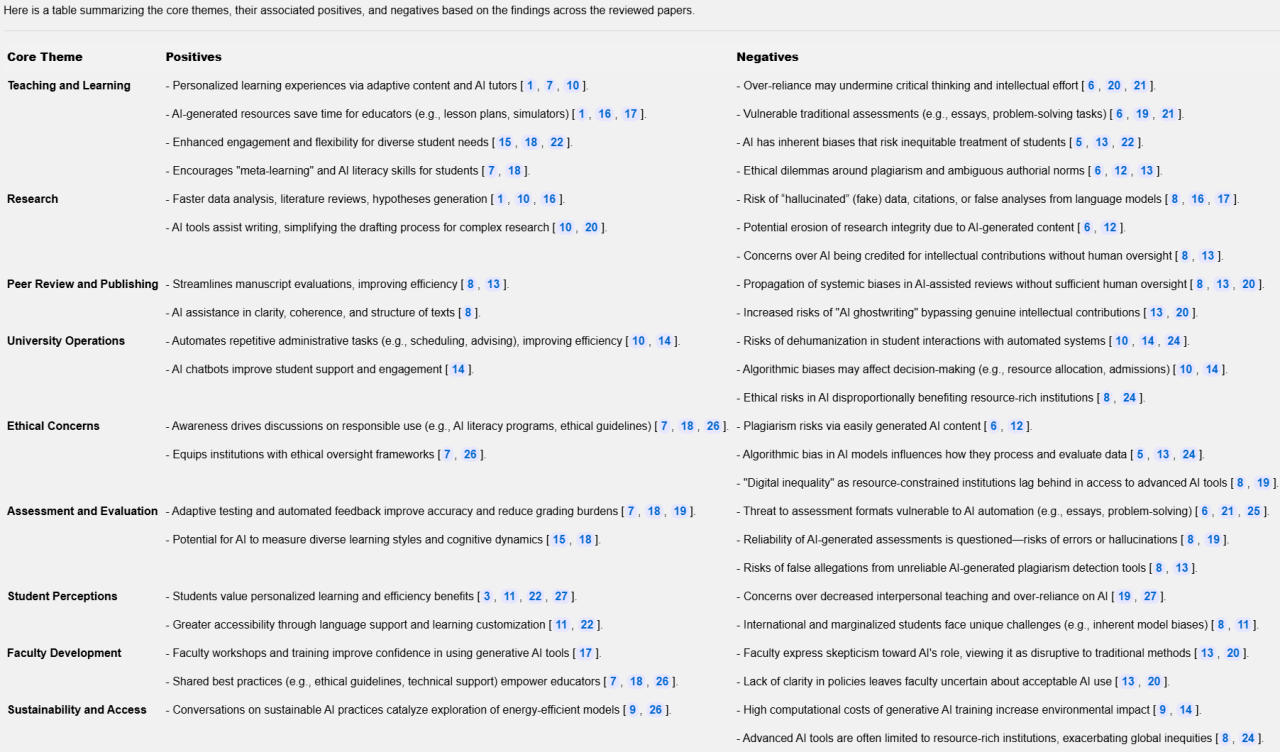
Elicit
Elicit can help speed up exploratory (but not systematic) literature reviews thanks to using advanced AI semantic search to identify relevant papers without the need for comprehensive or exact keywords. Like any tool that relies on searching data, it is limited by what it can access, and is generally not as comprehensive as Undermind.
It also includes LLM functionality to extract key information and/or summarise from retrieved articles as well as PDFs the user uploads and present in a table, columns include the title, abstract summary, main findings, limitations etc. The interface is very user-friendly and paid plans permit custom table columns for AI content, here's a screenshot of some options available in the free plan (limited to 4 columns):
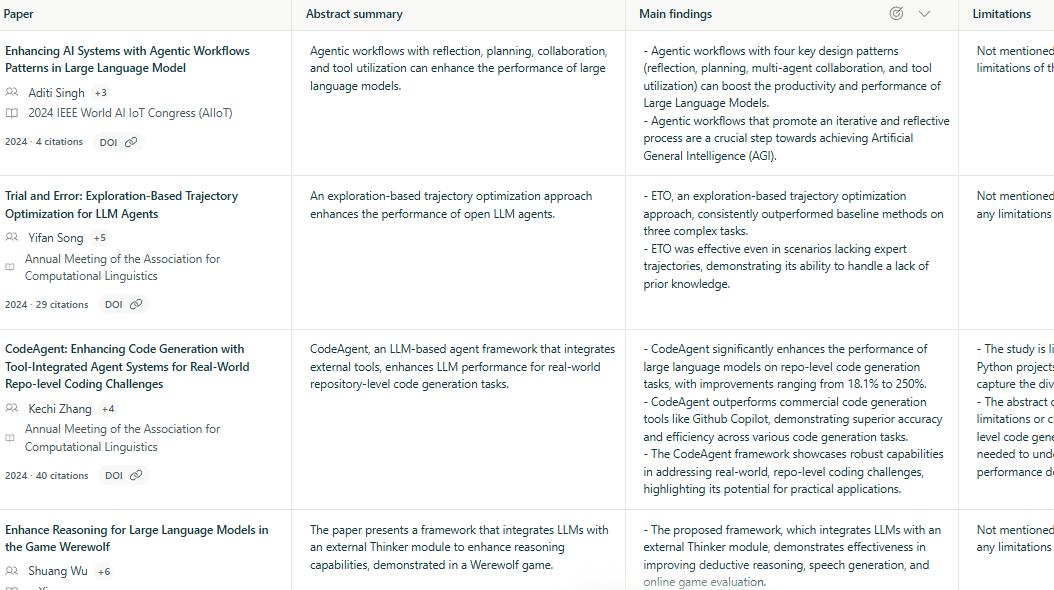
As with any LLM, results of such summaries or information retrieval cannot be relied upon and should only be seen as a starting point. The time saving may well be worth it at least for exploring a new research area.
Scite AI
Scite is similar to Elicit in terms of LLM-enhanced semantic searching to identify papers (again, limited to the scholarly databases it has access to). While it uses LLM technology to summarise a collection of papers from the search results, rather than being able to summarise key findings, limitations etc. like Elicit, it provides a view with short direct quotations from the paper along with direct links to the paper itself. In some cases this may be more useful than a potentially flawed LLM summary.
The main value offering of Scite is that it incorporates a limited evaluation of the nature of citation statements, to help a researcher get a quicker feel for the extent to which authors who cite a particular paper are supporting or critiquing it:
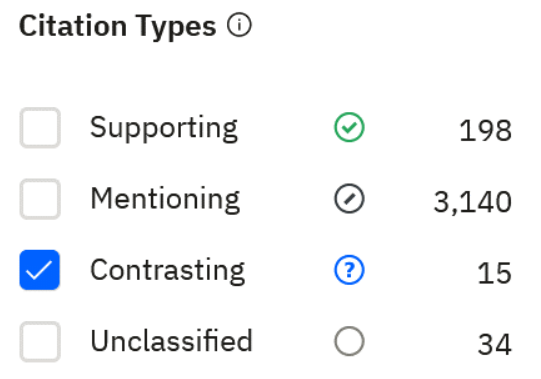
In reality, the overwhelming majority of citations are neutral and so the benefit is marginal – again, it could well be worth it in certain contexts to help accelerate discovery.
As with all AI-enhanced literature search tools, the value is far higher when exploring a new research area to speed up a researcher’s engagement with the literature. Identifying contrasting citations in particular can be extremely helpful to accelerate critical engagement with past studies or authors.
'Interrogating Papers'
The more explicit and targeted your prompts when ‘interrogating’ any specific academic text, the better. And this can be done just as well if not better with advanced LLMs (e.g. the o series from Open AI) if you have the papers already. Simply asking, “Please summarise the attached paper” will inevitably result in some data loss which may or may not be a problem depending on what you’re after. Something more targeted like “Please summarise the methodology in the attached paper along with stated limitations” (Important: remember to check Publisher Policies about whether publications are permitted to be shared with a generative AI application) is much more helpful because the scale of text needed to process is much smaller, and that also gives you more tokens to play with for follow up dialogue.
A useful way to experiment is by using an advanced LLM to interrogate with one of your own papers. This is an output you personally spent a lot of time on and you know it inside out, so you can easily verify how good its summaries or extractions are. This can then help you create prompt templates for other papers for more useful paper interrogations in future.