AI has the potential to analyse data fast and release valuable information that it could otherwise take human beings decades to detect. For companies the advantages are manifold. From detecting fraudulent transactions to improving customised shopping experiences; from operational automation to providing real-time assistance; from accelerating the recruitment process to driving customer engagement, loyalty and sales – deep learning has the power to transform business outcomes across almost every sector.
But if the advantages are clear, the risks are perhaps less so – as Apple found out to its cost. The Apple credit card hit the headlines for all the wrong reasons late in 2019, when users noticed major discrepancies between the credit lines offered to women and men. In some instances, married couples with similar incomes reported that the husband was eligible for several times more credit than the wife. Amid the furore and accusations of sexism, Wall Street announced it would pursue an enquiry to establish whether the Apple credit card was in breach of financial law. In response, Apple and partner, Goldman Sachs, insisted gender had not featured in the data set used to determine credit scorings. The problem, however, is that algorithms can still discriminate on any variable, even if they’ve been programmed to be “blind” to it. An algorithm that discounts gender, for instance, can still draw on data inputs that correlate to gender – shopping patterns, or the type of computer they use and so on.
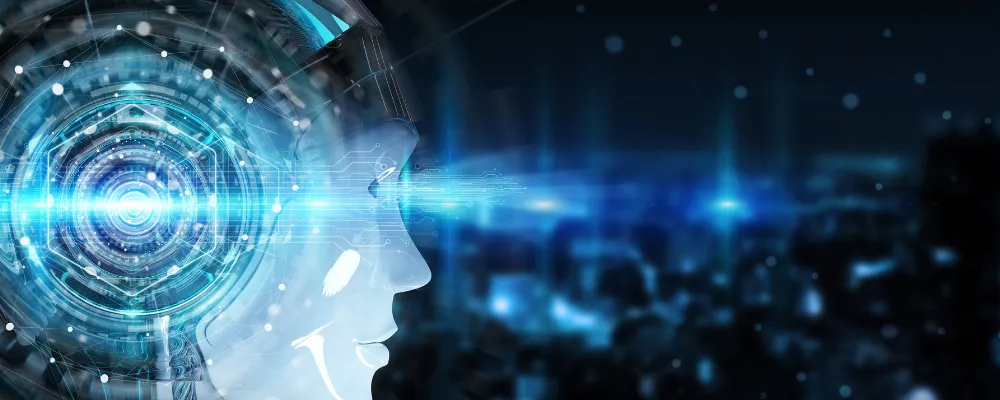
Worse still, by stripping out something as critical as gender, Apple had made it all the more difficult to identify any bias surrounding that very variable, and to take action to prevent or reverse it. Put crudely, the lesson for Apple was: garbage in, garbage out. The quality of all of the data you put into your algorithm, will determine the quality of what you get out of it.
And the Apple story isn’t unique.
Major league players like Amazon, Google and IBM have all had their fair share of AI embarrassments recently. There have been “misogynist” hiring algorithms, politically incorrect autocompletes and facial recognition technology, unintentionally skewed for gender and ethnicity. Perhaps the most calamitous of machine learning “fails” in the last few years can be credited to Microsoft chatbot, Tay – an experiment in building conversational intelligence using AI. Launched on Twitter on March 2016, Tay was designed to learn from interaction with human users, the idea being that the more the bot chatted, the more it would learn to engage people through “casual and playful conversation.”
Sadly for Microsoft, Twitter users almost immediately started sending its bot racist and misogynistic tweets which Tay immediately repeated back to other users, earning Microsoft unwanted epithets from the watching media. In total, Tay managed to send 96,000 tweets in 24 hours, many of them copied by users who had told the bot to “repeat after me.” The company closed Tay down the day after its launch having discovered two important lessons; the same lessons that Apple would go on to learn a few years later. First, to paraphrase the AI adage, what you put in with machine learning is essentially the same as what you get out: garbage in means garbage out. And secondly, if things go wrong with your algorithms, AIs and bots, it’s your reputation on the line.
As companies increasingly become reliant on algorithms to make critical decisions about customers and employees, the risks to things like corporate image and customer goodwill are only set to increase.
So what can they do to mitigate these risks while still reaping the huge advantages of deep learning to their business?
A key part of this puzzle revolves around the quality of the data that is used by algorithms to generate information. Maintaining the right kinds of data sets and mastering the techniques to ensure that the information is on point, clean and relevant is critical. Of course, as the technology itself evolves, so too do the techniques and methods for managing it. So there is a critical need to stay abreast of innovation in this space.
Data governance too – knowing what kind of data can be legally used – is of vital importance. And this doesn’t come without its challenges. Companies often anonymise data to keep within the limits of the law; but the very act of anonymising information can restrict or devalue the insights that a company needs to glean. So there are complex trade-offs between compliance and quality that also need to be navigated.
Perhaps most importantly, companies need to think critically about AI itself and resist the temptation to see data science as some kind of panacea or fix-all to be used indiscriminately in their business. Understanding the big picture and having the ability to distinguish the value of AI as a solution is key to determining whether it is the optimal solution or not. That means appreciating its limitations as much as its potential.