Richard Bradley’s written a new book about decision theory. We decided to ask him some questions about it.
Q: For the uninitiated, can you briefly explain the aims and methods of decision theory?
A: Decision theory aims both to describe how agents make decisions and how they should do so: what judgements they should make, what criteria they should employ and what rules they should follow. Both descriptive and normative decision theory draws on a mixture of mathematical, conceptual and empirical tools, though in differing proportions, in order to develop answers to these questions.
The central claim of normative decision theory is that we should choose in such a way as to maximize the expected benefit of our actions. Suppose you have to decide whether or not to buy fire insurance for your house. First you should consider what the costs and benefits of buying and of not buying would be in each possible circumstance. For instance, one salient consideration is that if there is a fire, your house will burn down. But if you have insurance, you will be compensated for the loss. On the other hand, if there is no fire, but you have bought insurance, then you will have spent a lot of money on premiums for no gain. Second you should assess how probable it is that there will be a fire. Finally, for each action, you should multiply your assessment of its costs and benefits in case of a fire by the probability of a fire, do the same for the case of no fire and then add up the results of the two cases. This will give you a numerical value for the expected benefit of acting. In practice if you think a fire is not extremely unlikely and the premiums are affordable, buying insuring will be sensible.
Q: What is Bayesianism, how does it relate to decision theory and to your own work?
A: Bayesianism is a wide-ranging doctrine about how to handle uncertainty that is influential in both decision theory and epistemology. It can be captured by three main claims regarding what to believe, how to revise beliefs and how to act.
- Believing: A rational agent should assign a precise probability to each possible state of the world and adopt this as her degrees of belief in its truth.
- Learning: A rational agent should revise her degrees of belief by conditionalising on the evidence that she gleans from experience, i.e. by adopting as her new beliefs, upon acquiring evidence E, her former conditional degrees of belief given that E.
- Deciding: A rational agent should choose the action with maximum expected benefit, where these expectations are calculated using her degrees of belief.
Not every Bayesian accepts all three claims, or indeed any in precisely the way I formulated them, but they jointly capture the version of it that serves as a foil to the discussion of uncertainty in my book.
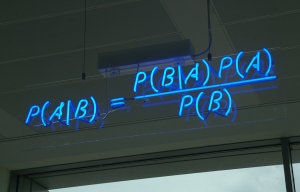
Bayes’ Theorem (in neon lights). Image credit: mattbuck/CC BY-SA 3.0
Q: You describe your own approach as decision theory with a human face. Does the “with a human face” indicate a difference in degree or a difference in kind from other forms of decision theory?
A: The approach that I develop in the book is best regarded as extending Bayesian theory into new domains. Bayesian decision theory is, in my opinion, the correct theory for a certain class of situation, namely one in which the agent is aware of all possible contingencies and is able to make a precise assessment of how probable and how desirable each is. The problem is that many situations are not like this! We often find ourselves unable to reach a judgement on relevant aspects of the decision problem we face, perhaps because of lack of information, perhaps because of restrictions on time or mental resources, or perhaps because we face conflicting considerations. In such situations it is reasonable to suspend or withhold judgement, at least partially. In the book I examine how agents should manage such situations: how they should represent their uncertainty, how they should revise their judgements in the light of experience and, of course, how they should make decisions. This takes us into territory where Bayesian theory is of limited applicability and where interesting questions arise regarding the rationality requirements on “bounded” agents facing severe uncertainty.
Q: What do you see as the implications of your work for other areas of philosophy?
A: Decision theory is closely connected to a number of other areas in philosophy, and indeed other academic disciplines, and so, not surprisingly, the book has implications for them. The most prominent one perhaps is epistemology. A central theme of the book is that a single probability measure on states of the world is inadequate as a representation of the uncertainty that we face. One of the richer representations of our epistemic situation that it explores, namely in terms of sets of probabilities, is already the subject of lively discussion in philosophy, economics and statistics under the banner of “imprecise probability’”. The book contributes to this discussion by examining not only the foundations and implications of this view, but also its limitations. In response to the latter a further enrichment is proposed, that draws on second-order judgements, such as reliability and confidence, regarding first-order probabilistic judgments to make sense of the fact that we often treat numerically identical probability judgements differently. Compare, for instance, betting on how a coin lands in a situation in which you know nothing at all about the coin to one in which you have had an opportunity to toss it numerous times and have observed that it lands heads about half the time. In both situations you may assign a probability of one half to the coin landing heads on the next toss, but how much faith you would put in this judgement would be quite different.
Q: How about for other, non-academic, areas of life?
A: The problem of making decisions under conditions of uncertainty is of course one of immense practical importance and I would hope that the lessons of the book will find wide application to policy making. This is especially true of areas where we face quite severe uncertainty, such climate and financial policy making. For these domains the book has a clear message. Firstly, that it is crucial to recognise and properly represent the uncertainty that is faced. Secondly that a wide range of strategies for dealing with it may be appropriate depending on the circumstances; including suspending judgement and delaying a decision, or reaching a provisional judgement for the immediate purposes of decision making, or employing a decision rule that draws on a wider set of considerations than just expected benefit, such as flexibility or security or robustness. Finally, that policy makers should calibrate their decisions, on the one hand to what is at stake in the decision and, on the other, to how confident they are in the judgements that they are applying to reach their decisions. When a lot is at stake and information sparse, then they should act more cautiously, for instance, than when little is at stake and/or their judgements are based on robust and plentiful evidence.
Richard Bradley is Professor of Philosophy in the Department of Philosophy, Logic and Scientific Method. In addition to decision theory, he is interested in the foundations of economic and social theory. Decision Theory with a Human Face will be published later this year, a working draft is available on Richard’s website.
Featured image credit: Mark Bernard